In the rapidly advancing field of precision medicine, where treatments are tailored to an individual’s genetic makeup, a glaring issue remains largely unaddressed—the lack of diversity in genomic research. The vast majority of genomic data used in medical studies comes from individuals of European descent, leaving large segments of the global population underrepresented. This disparity not only limits scientific understanding but also creates inequities in healthcare outcomes. Recent advancements in machine learning and efforts to diversify genomic datasets are poised to bridge this gap. However, without a concerted push for inclusivity, the promise of precision medicine risks becoming a privilege rather than a universal benefit.
Table of Contents
ToggleThe Impact of Ancestral Bias on Healthcare
Genomic data forms the foundation of precision medicine. By analyzing genetic markers, scientists can identify disease risks, develop targeted therapies, and customize drug treatments for individuals. However, when these datasets are disproportionately derived from one ancestral background, the results skew toward that population, leaving others at a disadvantage.
For instance, genome-wide association studies (GWAS) have identified thousands of genetic variants linked to diseases, but over 80% of participants in these studies are of European descent. This means that predictive models and medical treatments based on these studies are less reliable for individuals from African, Asian, Latino, or Indigenous populations. A study published in Nature Communications highlights how this lack of representation leads to misdiagnoses, improper dosages, and reduced effectiveness of treatments for non-European groups. Diseases such as sickle cell anemia, which predominantly affects African populations, and certain cancers that are more prevalent in Asian and Hispanic populations, are often under-researched due to this imbalance.
The Efforts to Diversify Genomic Data
Recognizing the problem, researchers and organizations have launched initiatives aimed at making genomic datasets more inclusive. The Human Pangenome Reference Consortium is one such effort, seeking to create a more representative reference genome by incorporating sequences from individuals of diverse ancestries. Unlike the current human genome reference, which is primarily built on data from a small number of individuals, this initiative aims to reflect the full genetic diversity of human populations.
Other projects, such as the All of Us Research Program by the National Institutes of Health (NIH), are working to collect genomic data from historically underrepresented groups. The goal is to ensure that precision medicine benefits everyone, regardless of their ethnic or genetic background. These efforts, while promising, are still in their early stages and face challenges related to funding, ethical concerns, and logistical complexities.
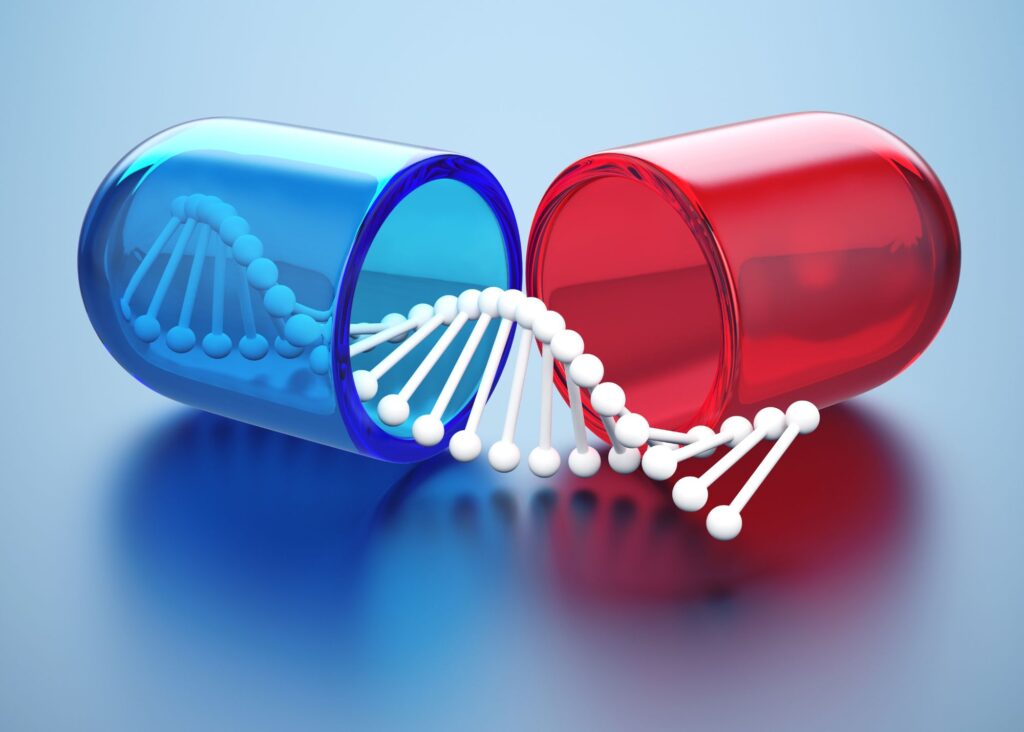
How Machine Learning Can Help (and Hurt)
Artificial intelligence and machine learning are revolutionizing genomic analysis. However, these technologies are only as good as the data they are trained on. If machine learning models are trained on biased datasets, they perpetuate the very disparities they aim to resolve.
For example, facial recognition technologies have been widely criticized for their racial biases (MIT Media Lab Study). Similarly, in genomics, biased datasets can lead to erroneous predictions about disease risks in non-European populations. To counteract this, researchers are developing fairness-aware machine learning techniques that mitigate bias at different stages of data processing:
- Preprocessing: Adjusting datasets to balance representation before training models.
- Inprocessing: Incorporating fairness constraints during the model training process.
- Postprocessing: Modifying model outputs to ensure more equitable results.
These techniques are essential for ensuring that genomic research leads to accurate and fair medical applications for all populations. Without them, AI-driven precision medicine could inadvertently reinforce existing healthcare disparities rather than eliminate them.
The Ethical and Logistical Challenges of Inclusive Genomics
Expanding genomic diversity is not just a scientific challenge—it is an ethical one. Historically, marginalized communities have been wary of genetic research due to past abuses, such as the Tuskegee syphilis study and the unauthorized use of Henrietta Lacks’ cells. Building trust with these communities requires transparency, informed consent, and safeguards against the misuse of genetic data.
Additionally, there are logistical hurdles. Recruiting diverse participants for genomic studies is costly and time-consuming. Many underrepresented populations have limited access to healthcare facilities, making sample collection difficult. Furthermore, the question of data sovereignty arises—who owns and controls the genetic information collected from different populations? Ethical frameworks must be in place to ensure that genetic data is used responsibly and that communities benefit from research conducted on their genetic information.
The Path Forward: Making Precision Medicine Truly Inclusive
The field of precision medicine is at a crossroads. On one hand, the technology and knowledge exist to develop highly personalized treatments that could revolutionize healthcare. On the other, the exclusion of diverse populations from genomic studies threatens to turn these advancements into tools of inequality.
To move forward, multiple steps must be taken:
- Increase Funding for Diverse Genomic Research: Governments, private institutions, and biotech companies must prioritize funding for initiatives that expand representation in genomic datasets.
- Enhance Ethical Standards and Regulations: Researchers must adhere to strict ethical guidelines to ensure genetic data is used responsibly and with informed consent from participants.
- Leverage AI to Reduce Bias: The development of unbiased machine learning algorithms is crucial for equitable precision medicine.
- Engage Communities in Research: Building trust with historically underrepresented groups is essential for diversifying genomic research. Outreach programs, education initiatives, and community involvement can foster participation.
Conclusion
Precision medicine holds incredible promise for the future of healthcare, but it cannot truly succeed without addressing ancestral bias in genomic data. Without diverse representation in genetic research, entire populations risk being left behind in the advancements of modern medicine. Through equitable machine learning, ethical research practices, and committed funding, the scientific community can ensure that precision medicine benefits everyone—not just those with European ancestry. The challenge ahead is significant, but the potential rewards—a healthcare system that works for all—are worth the effort.