Antibiotic resistance poses a significant global health threat, rendering many traditional antibiotics ineffective and leading to increased mortality and healthcare costs. The conventional drug discovery process is often slow and costly, struggling to keep pace with the rapid evolution of resistant pathogens.
Artificial intelligence (AI) has emerged as a transformative tool in drug discovery, capable of analyzing vast datasets to identify potential antibiotic candidates more efficiently than traditional methods. By leveraging machine learning algorithms, researchers can expedite the identification and development of novel antibiotics, addressing the urgent need for effective treatments against resistant bacteria.
Table of Contents
ToggleRecent Breakthroughs in AI-Driven Antibiotic Discovery
- Discovery of Halicin: In 2020, researchers at the MIT Jameel Clinic, including Professors Regina Barzilay and James J. Collins, utilized a deep learning model to identify halicin, a compound effective against various antibiotic-resistant bacteria, including Clostridioides difficile and Acinetobacter baumannii. Halicin operates by disrupting the bacteria’s ability to maintain an electrochemical gradient across their cell membranes, a mechanism distinct from traditional antibiotics.
- Development of Abaucin: In 2023, the same MIT research team employed AI to discover abaucin, a narrow-spectrum antibiotic targeting Acinetobacter baumannii, a pathogen identified by the World Health Organization as a critical threat. Abaucin’s unique mechanism involves inhibiting lipoprotein transport in the bacteria, showcasing AI’s potential to identify specialized antibacterial agents.
- AI-Designed Antimicrobial Peptides: Recent advancements have seen AI models facilitating the rapid design of antimicrobial peptides (AMPs) with desired properties. For instance, the AMP-Designer framework enabled the creation of novel peptides with broad-spectrum potency against Gram-negative bacteria. This approach demonstrates AI’s efficiency in accelerating drug discovery processes.
Mechanisms of AI in Antibiotic Discovery
- Data Analysis and Pattern Recognition: AI algorithms can process extensive chemical and biological datasets to identify patterns and predict antibacterial activity, expediting the identification of promising compounds. For example, deep learning models have been trained to recognize molecular structures with potential antibiotic properties.
- Predictive Modeling: Deep learning models can predict the efficacy and toxicity of potential antibiotics, reducing reliance on trial-and-error approaches. This predictive capability allows researchers to focus on the most promising candidates, streamlining the drug development pipeline.
- Generative Design: AI can generate novel molecular structures with desired antibacterial properties, expanding the chemical space for potential antibiotics. For instance, AI-driven generative models have been used to design new antibiotics effective against drug-resistant pathogens.
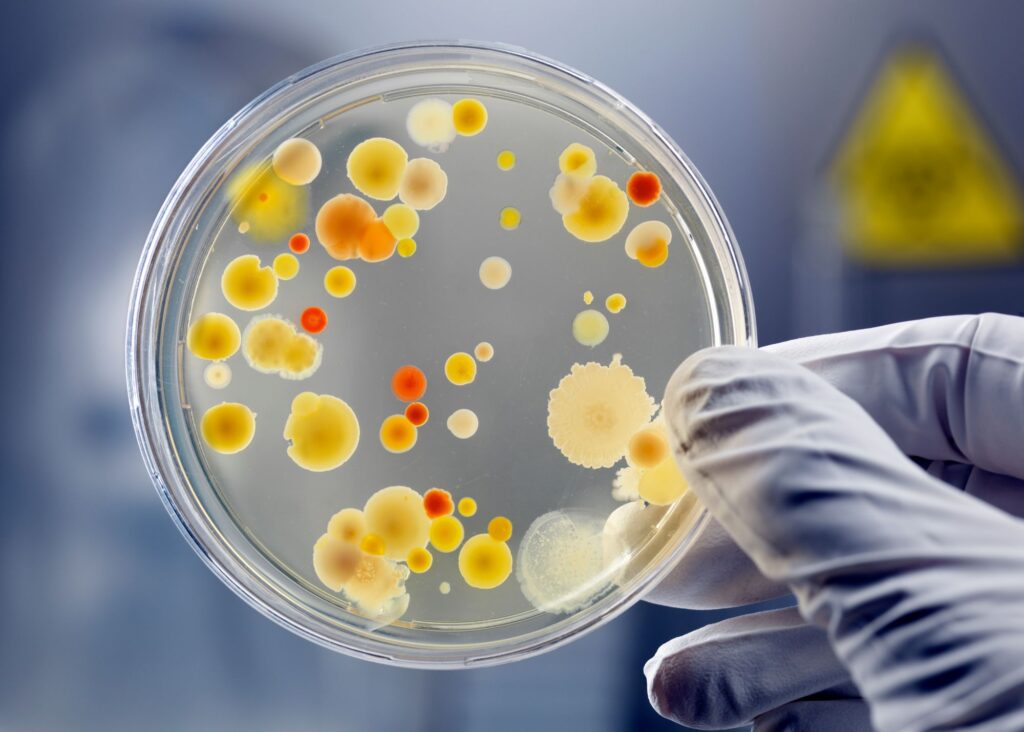
Advantages of AI in Antibiotic Discovery
- Efficiency: AI accelerates the drug discovery process by rapidly analyzing extensive datasets, enabling the swift identification of potential antibiotic candidates. This efficiency significantly reduces the time required to bring new antibiotics from conceptualization to clinical application.
- Cost-Effectiveness: By streamlining the early stages of drug development and reducing the reliance on trial-and-error methods, AI lowers research and development costs. This economic advantage is crucial in addressing the financial challenges associated with traditional antibiotic discovery.
- Precision: AI’s ability to design antibiotics with specific mechanisms of action enhances the precision of targeting bacterial pathogens. This targeted approach minimizes off-target effects and reduces the likelihood of adverse reactions, improving patient outcomes.
Challenges and Considerations
- Data Quality and Availability: AI models depend on high-quality, comprehensive datasets to make accurate predictions. However, the scarcity of such datasets, particularly for rare or emerging pathogens, poses a significant challenge. Ensuring data accuracy and representativeness is essential for reliable AI-driven antibiotic discovery.
- Integration with Experimental Validation: While AI can predict potential antibiotic candidates, experimental validation remains indispensable. Bridging the gap between in silico predictions and in vitro or in vivo testing is crucial to confirm the efficacy and safety of AI-designed antibiotics.
- Regulatory and Ethical Aspects: The integration of AI in drug discovery introduces regulatory and ethical considerations. Ensuring transparency in AI algorithms, addressing potential biases, and establishing robust regulatory frameworks are vital to gain public trust and facilitate the adoption of AI-designed antibiotics.
Future Prospects
- Collaborative Efforts: Fostering interdisciplinary collaboration between AI experts, microbiologists, pharmacologists, and regulatory bodies is essential to harness AI’s full potential in antibiotic discovery. Such collaborations can lead to innovative solutions and expedite the development of effective antibiotics.
- Continuous Learning Models: Developing AI models that continuously learn from new data can enhance predictive accuracy over time. This adaptability allows AI systems to stay abreast of evolving bacterial resistance patterns, ensuring the ongoing effectiveness of discovered antibiotics.
- Personalized Medicine: AI holds the potential to design antibiotics tailored to individual patient’s microbiomes, ushering in an era of personalized antimicrobial therapy. This approach can optimize treatment efficacy and minimize the disruption of beneficial microbiota.
Conclusion
AI is revolutionizing antibiotic discovery by enhancing efficiency, reducing costs, and increasing precision. The breakthroughs achieved underscore AI’s potential to address the global antibiotic resistance crisis effectively.
Continued investment in AI research and development is imperative. Policymakers, researchers, and industry stakeholders must collaborate to integrate AI innovations into mainstream antibiotic development pipelines, ensuring the timely delivery of effective treatments to combat resistant bacterial infections.
By embracing AI’s capabilities and addressing the associated challenges, the biotech industry can usher in a new era of antibiotic discovery, safeguarding public health against the looming threat of antibiotic resistance.