Table of Contents
ToggleAbstract
This report explores the transformative impact of generative artificial intelligence (AI) on protein design. By leveraging advanced computational models, generative AI offers unprecedented capabilities in creating novel protein structures and sequences, significantly enhancing the efficiency and scope of drug discovery and therapeutic development. This report provides an overview of traditional protein design methods, the integration of generative AI, and detailed insights into leading AI platforms and their applications. The findings underscore the potential of generative AI to revolutionize biotechnology and medicine.
Introduction
Protein design is a critical area of research with far-reaching implications in biotechnology, medicine, and synthetic biology. Traditionally, protein design has relied on understanding and manipulating natural protein structures to develop new drugs and therapies. However, these conventional methods are limited by their dependency on existing protein templates and the complexity of protein folding.
The advent of generative AI has introduced a paradigm shift in protein design. Generative AI employs machine learning algorithms to create new protein structures and sequences from scratch, going beyond the natural protein landscape. This capability opens new avenues for designing proteins with specific, programmable properties, which can lead to breakthroughs in drug discovery, enzyme engineering, and therapeutic development.
The purpose of this report is to provide a comprehensive overview of how generative AI is being applied to protein design, highlight key methodologies and models, and discuss the implications of these advancements for the future of biotechnology and medicine.
Literature Review
Traditional protein design methods primarily involve the use of X-ray crystallography, nuclear magnetic resonance (NMR) spectroscopy, and cryo-electron microscopy to determine protein structures. These techniques, while powerful, are time-consuming and resource-intensive. Additionally, the design process often involves iterative cycles of mutation and selection, which can be laborious and inefficient.
In contrast, generative AI models offer a more efficient approach by using machine learning algorithms trained on large datasets of protein sequences and structures. These models can generate new protein sequences that fold into desired structures, predict protein-protein interactions, and optimize protein functions. Some of the notable AI models and platforms in this field include:
- NVIDIA BioNeMo Platform: NVIDIA’s BioNeMo platform utilizes generative AI to enhance drug discovery by offering models for protein structure prediction, sequence generation, and molecular optimization. It is designed to reduce the need for physical experiments, thereby accelerating the drug development process. BioNeMo includes tools like MolMIM for small molecule generation and OpenFold for protein structure prediction, which are being used by companies like Amgen and Recursion.
- MIT’s FrameDiff Model: FrameDiff is a generative AI model developed by MIT that constructs protein backbones using a process called SE(3) diffusion. This model has applications in vaccine design and enzyme engineering, demonstrating the potential of AI to create new protein structures with specific functions.
- Generate’s Chroma Model: Chroma is a generative AI model from Generate that focuses on de novo protein design. It uses diffusion models to generate novel proteins with programmable properties, expanding the functional repertoire beyond natural proteins. Chroma has shown success in creating proteins that are experimentally validated to fold correctly and possess desirable biophysical properties.
These advancements in generative AI are revolutionizing protein design by making it possible to create proteins with unprecedented precision and efficiency. This new approach not only accelerates the discovery of new drugs and therapies but also expands the potential applications of synthetic biology.
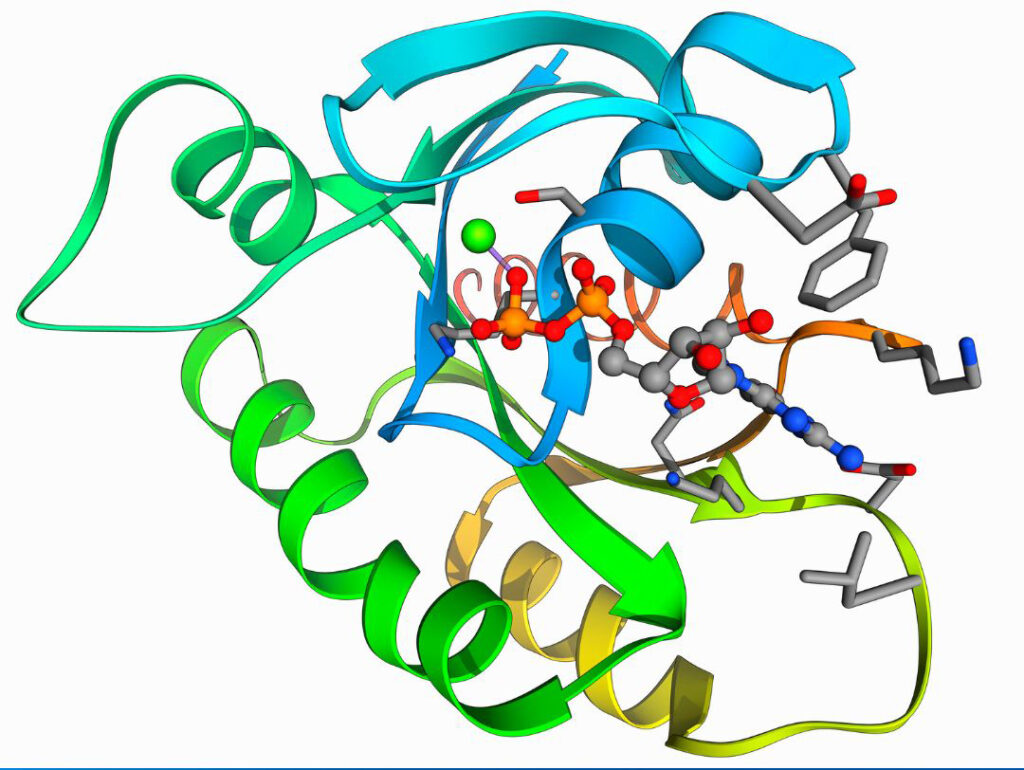
Methodologies in Generative AI for Protein Design
NVIDIA BioNeMo Platform
The NVIDIA BioNeMo platform stands out as a transformative tool in drug discovery and protein design. It integrates a suite of generative AI models that offer new computational methods to streamline and enhance research and development processes in the pharmaceutical and biotechnology sectors.
Components and Capabilities:
- Pretrained Biomolecular AI Models: BioNeMo includes models for protein structure prediction, sequence generation, molecular optimization, generative chemistry, and docking prediction. These models, such as MolMIM for small molecule generation and OpenFold for protein prediction, provide researchers with robust tools to innovate within drug discovery and protein engineering.
- Cloud-based Services: The platform offers cloud APIs that allow drug discovery teams to integrate AI seamlessly into their workflows, enabling virtual experiments that reduce the need for time-consuming and costly physical experiments. This integration is facilitated through partnerships with cloud service providers like AWS HealthOmics, enhancing accessibility and scalability.
- Generative Models: BioNeMo’s models, including ESM-1nv and ESM-2 for protein property predictions and MegaMolBART for molecular generation, are designed to handle large-scale biological datasets. These models help predict various protein properties, such as stability and solubility, and optimize molecular structures for desired therapeutic outcomes.
Applications and Impact:
- Drug Discovery: BioNeMo is used by over 100 companies, including Astellas Pharma, Insilico Medicine, and Recursion, to accelerate drug discovery processes. These companies leverage BioNeMo’s AI capabilities to develop new therapeutics, optimize drug candidates, and predict protein-ligand interactions, significantly reducing the development timeline and enhancing precision in drug design.
- Customization and Scalability: Researchers can customize and fine-tune BioNeMo models to their specific needs, allowing for targeted therapeutic development. This capability is crucial for addressing complex biological challenges and advancing personalized medicine.
MIT’s FrameDiff Model
The FrameDiff model, developed by researchers at MIT, represents a significant advancement in generative AI for protein design. This model constructs protein backbones through a process known as SE(3) diffusion, which involves learning to generate new protein structures by manipulating frames in a spatial context.
Technical Details and Applications:
- SE(3) Diffusion: FrameDiff uses stochastic calculus on Riemannian manifolds to learn probability distributions that connect the translation and rotation components of protein frames. This method allows the model to create accurate and novel protein structures that can be experimentally validated.
- Applications in Biotechnology: The model’s ability to generate new protein backbones has significant implications for vaccine design, enzyme engineering, and therapeutic protein development. By creating proteins that do not exist in nature, FrameDiff opens new possibilities for addressing various biomedical challenges.
Impact and Future Directions:
- Enhanced Protein Design: FrameDiff’s innovative approach to protein generation enables the creation of highly specific protein structures, which can be used in developing new vaccines and enzymes with improved efficacy and stability.
- Ongoing Research: Future research aims to expand FrameDiff’s capabilities to design side-chain configurations and other complex protein features, further enhancing its utility in synthetic biology and therapeutic development.
By integrating these advanced methodologies, generative AI platforms like NVIDIA BioNeMo and MIT’s FrameDiff model are revolutionizing the field of protein design, offering unprecedented precision and efficiency in developing new therapeutics and biotechnological applications.
Key Findings and Developments
NVIDIA BioNeMo Platform
The NVIDIA BioNeMo platform has made significant strides in generative AI for drug discovery and protein design. Key findings and developments include:
- Pretrained Models and Cloud Integration: BioNeMo offers a range of pretrained biomolecular AI models for tasks such as protein structure prediction, sequence generation, and molecular optimization. These models are integrated into cloud services like AWS HealthOmics, allowing researchers to conduct virtual experiments efficiently.
- High-Performance AI Models: The platform includes models like ESM-1nv and ESM-2, which are designed for protein property predictions, and MegaMolBART for molecular generation. These models can predict protein properties, optimize molecular structures, and facilitate drug discovery by reducing the need for extensive physical experiments.
- Industry Adoption: More than 100 companies, including Astellas Pharma, Insilico Medicine, and Recursion, use BioNeMo to enhance their drug discovery processes. These companies leverage BioNeMo’s capabilities to develop new therapeutics, optimize drug candidates, and predict protein-ligand interactions, significantly accelerating the development timeline.
- Customization and Scalability: BioNeMo allows researchers to customize and fine-tune models to meet specific needs, which is crucial for addressing complex biological challenges. This flexibility enhances the platform’s utility in developing personalized medicine and other advanced biotechnological applications.
Generate’s Chroma Model
Chroma model represents a breakthrough in generative AI for protein design. The model is designed to create novel proteins with programmable properties, advancing the field significantly:
- Generative Capabilities: Chroma uses a diffusion process that respects the conformational statistics of polymer ensembles and employs random graph neural networks to generate novel protein structures. This model can create proteins with specific shapes, symmetries, and functions, making it a powerful tool for protein engineering.
- Experimental Validation: Chroma has demonstrated its capability by generating proteins that express, fold, and exhibit favourable biophysical properties. Experimental characterization of 310 proteins showed that Chroma’s predictions closely match real protein structures, with atomistic accuracy in some cases.
- Open Source and Collaboration: Generate has made Chroma’s code and model weights available as open-source, encouraging broader research collaboration. This initiative aims to accelerate scientific progress in generative biology by allowing researchers to build on Chroma’s capabilities.
- Applications and Impact: Chroma’s ability to design novel proteins with targeted therapeutic properties holds significant potential for drug discovery and development. The model’s flexibility in handling various protein design challenges, from symmetry conditioning to semantic control, makes it a versatile tool in biotechnology and medicine.
Discussion
The integration of generative AI in protein design, as exemplified by NVIDIA’s BioNeMo, MIT’s FrameDiff and Chroma, marks a significant advancement in the field. These platforms not only enhance the efficiency of drug discovery but also expand the possibilities for creating novel proteins with specific, programmable properties.
Impact on Drug Discovery: Generative AI models like those in BioNeMo and Chroma enable researchers to conduct virtual experiments, reducing the reliance on physical trials. This approach accelerates the development of new therapeutics, potentially lowering costs and improving the speed at which new drugs reach the market.
Advantages Over Traditional Methods: Traditional protein design methods are often limited by the available natural protein templates and the complexity of protein folding. Generative AI overcomes these limitations by enabling the creation of entirely new protein structures, opening up new avenues for innovation in medicine and biotechnology.
Challenges and Limitations: Despite their potential, generative AI models also face challenges, including the need for extensive computational resources and the complexity of accurately predicting protein behaviour in vivo. Additionally, ethical considerations and regulatory challenges must be addressed as these technologies become more integrated into drug development pipelines.
Ethical Considerations: The ability to create novel proteins and potentially new biological entities raises ethical questions about the implications of such technologies. Ensuring responsible use and addressing potential risks, such as unintended consequences or misuse, is crucial for the continued advancement of generative AI in biotechnology.
By leveraging the strengths of platforms like BioNeMo and Chroma, researchers can explore new frontiers in protein design, paving the way for innovative treatments and applications in various fields, from medicine to materials science.
Future Directions
The field of generative AI for protein design is poised for significant advancements, promising to transform biotechnology and therapeutic development in numerous ways. Here are some key areas and future directions for this technology:
Expansion to New Biological Modalities: Generative AI models are expected to extend beyond protein design to include other biological modalities such as DNA and small molecules. This expansion will enable the design of more complex biological systems, potentially revolutionizing fields like gene therapy and synthetic biology.
Integration of Advanced Models: The integration of advanced models like DiffDock and ESMFold, which predict protein-ligand interactions and protein structures with high accuracy, is anticipated to enhance the precision and efficiency of drug discovery. These models will facilitate the development of novel therapeutics by accurately predicting how proteins interact with potential drug molecules.
Programmable and Modular Approaches: Generative AI platforms are increasingly incorporating modular and programmable approaches. Tools like Chroma from Generate exemplify this trend by allowing researchers to program proteins with specific properties using various conditioning techniques such as symmetry, shape, and natural-language prompts. This flexibility will accelerate the creation of tailored protein therapeutics and materials.
Ethical and Responsible AI Use: As generative AI becomes more integral to biotechnology, there is a growing emphasis on ethical considerations and responsible use. Guidelines and frameworks are being developed to ensure that AI-driven protein design is conducted safely and ethically, addressing potential risks and ensuring beneficial outcomes for society.
Collaborative and Open-Source Efforts: Open-source initiatives and collaborative research are expected to play a crucial role in advancing generative AI for protein design. By making tools and models like Chroma publicly available, researchers worldwide can contribute to and benefit from collective advancements, accelerating scientific progress and innovation in the field.
Conclusion
The integration of generative AI in protein design is revolutionizing the field by providing tools that enhance the precision and efficiency of drug discovery and protein engineering. Platforms like NVIDIA BioNeMo, MIT’s FrameDiff and Chroma are at the forefront of this transformation, enabling the creation of novel proteins with programmable properties and expanding the possibilities for therapeutic and biotechnological applications.
These advancements not only promise to accelerate the development of new drugs and therapies but also open up new frontiers in synthetic biology and materials science. As the technology continues to evolve, it will be essential to address ethical considerations and foster collaborative efforts to maximize the positive impact of generative AI on society.